
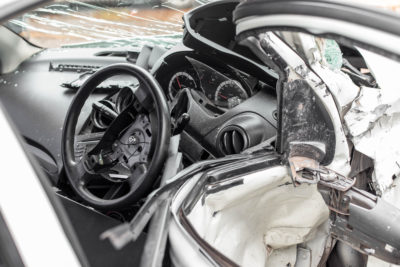
A FUTURE IN CRASH RESPONSE

Best Practices
By Keith Griffiths
A recent study incorporating crash data from 836 vehicles equipped with advanced automated crash notification (AACN) capabilities demonstrates that telemetry from these systems can be used to accurately predict the injury severity of vehicle occupants.
The research used an algorithm to analyze three types of data from each accident: crash factors, vehicle factors, and occupant factors (924 occupants in the 836 accidents in the study). The results were used to predict whether each vehicle occupant met the 20 percent or higher risk of having an Injury Severity Score (ISS) of 15-plus, the threshold for urgent transport to a trauma center.
According to the study’s findings, the methodology is successful in predicting occupants with a high likelihood of severe injury with a sensitivity of 63 percent and a specificity of 96 percent, when the gender and age of the vehicle occupants were known. Without information about age and gender, the sensitivity was 45 percent, while the specificity was 98 percent.
“Sixty-three percent sensitivity may not sound like an impressive number, but when you look at the experience over the last four to five decades, it is a significant improvement,” said Principal Investigator Dr. Stewart Wang, a trauma surgeon and Director, University of Michigan Program for Injury Research and Education (UMPIRE). “Even trained personnel are no more than 40–50 percent accurate in picking out patients with severe injuries.”
How AACN works
In most vehicles equipped with AACN capabilities, including all those using OnStar, which was the AACN technology reviewed in this study, the call goes to a call center, and the call center then separately contacts the PSAP. A panel of AACN and patient triage experts made recommendations on what telemetry information a vehicle should automatically transmit, although all information may or may not go to the call center and a subset that might typically be sent to the PSAP includes:
• Whether the crash included multiple impacts
• The vehicle’s change in velocity
• The principal direction of force
• Whether or not seat belts were in use
• The type of vehicle
This information offers 911 and EMS personnel three primary advantages when responding to a crash: faster notification of the crash; the exact location information for the accident; and telemetry data, which can be used to predict injury severity and injury patterns.
“The data we can get from AACN adds important information to the data points we can get in-field,” said Dr. Scott Sasser, Associate Director, International Programs for the Center for Injury Control at Emory University and the principal investigator for a new AACN training course. “This data can help us allocate the appropriate resources to the scene.”
AACN data going to Emergency Dispatchers
Systematically routing data to the 911 system should be the starting point in the process, according to experts at the International Academies of Emergency Dispatch (IAED).
“Emergency Dispatchers are the first place that AACN data bounces off,” said Dr. Jeff Clawson, IAED co-founder and inventor of the Fire, Police, and Medical Priority Dispatch Systems. “Having an actual score to predict injury severity is what is going to get people’s attention. Emergency Dispatchers want to know where they plug that in. That information needs to be taken in and formed in a structured protocol.”
The Academy has been working with OnStar for more than a decade. The Medical Priority Dispatch System (MPDS) Protocol contains two Chief Complaints—priority coded emergency events—involving motor vehicle collisions. Also, IAED provided a CBT lesson as a “Special Procedures Briefing” for Protocol 34: Automatic Crash Notification (ACN).
“We use Protocol 34, which is a protocol to help 911 capture this information when it is just an automatic crash notification,” Dr. Clawson said. “This was based on talking to people in the vehicle and hearing their report of occupants’ status and not telematics. Now, the telematics data can feather right into that existing protocol to help verify and add to what we know about occupants’ status and tease out what is important in a sea of data.”
The algorithm predictions provide information important to emergency dispatchers directing EMS response, and the IAED is developing the programming required to bring AACN data into its existing protocols and capture this data using ProQA software.
There is a balancing point in the amount of information that dispatch should receive, Wang said.
“If bad information is passed along to PSAPs or they receive too much data, it is like throwing sand in the emergency dispatch processes,” Wang said. “The expert committee tried very hard to say that when using AACN for the purposes of triage, you should go with a system that communicates high-risk for severe injury, like a red light or green light to the 911 system.”
The ultimate goal is saving lives, Sasser said.
“We want to look at whether AACN can help us lower morbidity and mortality with motor vehicle crashes,” he said.
Learn more about AACN
A new one-hour online training program is now available for EMS professionals interested in learning more about AACN technology and the implications of AACN crash data. This course covers current AACN research, biomechanics of crash injuries, how data can be used to predict injury severity, and ways to integrate this data into local 911 and EMS systems. The online training program is supported by the American College of Emergency Physicians (ACEP) and the National Association of EMS Physicians (NAEMSP), with funding from the National Highway Traffic Safety Administration (NHTSA).
Sasser notes that the training program keys into the growing relevance of automatic crash data to EMS.
“We need to know how the information supplied by the vehicle can identify injury patterns and also help with everything from resource allocation to destination decision-making,” he said.
See the training at www.aacnems.com.
References
Wang SC, Kohoyda-Inglis CJ, MacWilliams JB, et al. “Results of First Field Test of Telemetry Based Injury Severity Prediction.” https://www.acep.org/globalassets/support/innovatED_supportfiles/RESULTS-OF-FIRST-FIELD-TEST-OF-ELEMETRY-BASED-INJURY-SEVERITY-PREDICTIO.pdf (accessed Jan. 29, 2016).
More Articles
The Brave Conversations Method
How to stop avoiding conflict and start navigating it with confidence